#323 Revolutionizing SME Finance Management: Metric CTO on the AI Advantage in FinTech
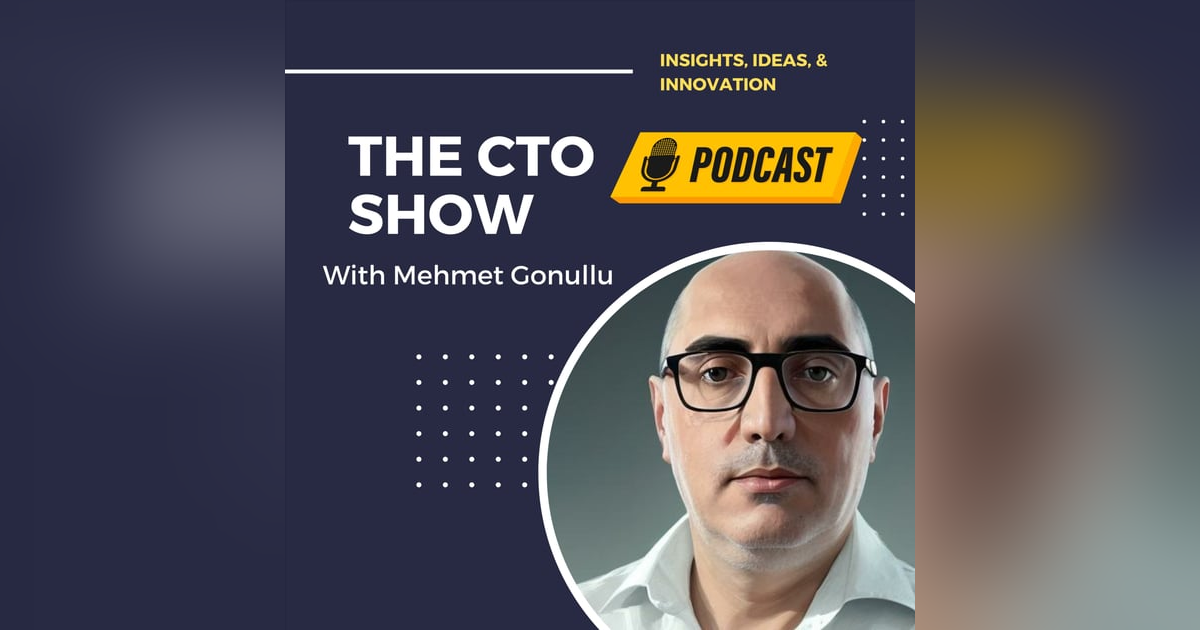
In this episode of the CTO show, host Mehmet is joined by Habiba, CTO and co-founder of Metric, who shares her rich background in AI and machine learning, transitioning from academia to the tech industry, and her current role at Metric.app.
Habiba discusses her commitment to leveraging AI for providing personalized financial management solutions to small and medium-sized businesses. With a vast experience spanning research in dynamic networks, postdoctoral work in historical trade routes, and roles in various countries and sectors including retail and government, Habiba emphasizes the importance of customer feedback in shaping Metric.
The conversation touches on the challenges of scaling in different geographic regions, potential of generative AI in FinTech, and Habiba's advice for aspiring tech entrepreneurs and professionals in the AI sphere.
More About Habiba:
Habiba is an applied machine learning and deep learning engineer with extensive experience across various sectors. Currently, she serves as a Data Scientist at the Department of Education in NSW, focusing on applying Large Language Models in the education space. Previously, she was an AI Cloud Solution Architect at Microsoft, enhancing customer adoption of machine learning through Azure services. She is also the CTO of Metric, a fintech startup designed to simplify financial management for SMEs and startups through technology-enabled accounting and insights into financial health.
https://www.linkedin.com/in/habibah
Special Promo Code For Our Audience:
Get 50% off on any Metric plan for 3 months
Promo code: CTO50
02:03 Habiba's Journey: From Academia to CTO of Metric
05:40 The Evolution of Metric: Empowering SMEs with AI
10:37 Customer Insights: Shaping Metric's Development
16:51 The Future of FinTech and AI: A Deep Dive with Habiba
21:05 Advice for Aspiring Data Scientists and Tech Entrepreneurs
30:42 Closing Thoughts and Where to Find More About Metric
[00:00:00]
Mehmet: Hello and welcome back to a new episode of the CTO show with Mehmet. Today I'm very pleased joining me Habiba. Habiba, she's the CTO and co founder of metric.app. Thank you very much for being with us here today Habiba. The way I love to do it is I keep it to my guests to [00:01:00] introduce themselves, to tell us a little bit more about their background and what they are up to.
Mehmet: So the floor is yours.
Habiba: Sure. Thank you so much, Mehmet. It's so nice to meet you and to talk to you here today. So, yes, my name is Habiba. I am the CTO of Metric, um, and I've been, uh, with the, uh, with the team since the very beginning. As you mentioned, I'm one of the co founders. My background is in AI and machine learning, um, in computer science.
Habiba: I had been, uh, working in this field for more than two decades now. And, uh, as a, as a CTO, I oversee our tech team and our, uh, the, uh, The couple of apps that we have built, which is one of them is our Android app and a web app. And now we are launching our iOS app as well. The, uh, our, uh, the application that we are building is for small and medium sized businesses, uh, to, uh, to manage their finances and, uh, and to give ins, uh, insights to them to help them grow their businesses.
Habiba: So, yeah, this is, uh, what I do. Um, a bit of, uh, if you want, I can start with my background, uh, [00:02:00] myself, uh, you know, yeah,
Mehmet: actually what I'm interested in, I know if you can tell me a little bit more, I know that you have done some transition from, you know, doing research and kind of academia to where you are today, you know, uh, with your journey.
Mehmet: So if you can like highlight a little bit about that journey as well.
Habiba: Sure. Uh, so starting from the start, I have, uh, uh, I'm a big math enthusiast, so this is where my, all my inspiration started from, uh, from, you know, like trying to do something in mathematics, um, which transitioned into computer science.
Habiba: I got my undergraduate in computer science from, uh, from, uh, from Pakistan, where I am originally from. And then I moved to the US to the University of Illinois, Chicago, where I went for my grad school. I did my PhD in computer science from there in, um, and basically the topic that I was working on was finding, um, uh, uh, influential individuals in dynamic networks.
Habiba: So it was basically a network application of. [00:03:00] of data driven method or methodology. Um, after my PhD, I went to Germany to do a postdoc, um, where I work with, uh, in a, in an, in a European research council funded project on, um, uh, on the, uh, uh, on the times of, uh, when, uh, uh, when the, uh, colonial invasions of the Caribbean.
Habiba: So it was a really interesting application of, uh, uh, Uh, of machine learning and data science to build methods around how, um, trade routes, uh, and how population dispersion happened during colonization of the Caribbean, um, islands. Um, I personally enjoy, uh, this, uh, side of computer science where I am applying technical methodology to, uh, to applications in real world.
Habiba: From, um, from there onwards, I moved to, uh, to, to more industrial setting, uh, where I worked as a data scientist for auto, which is an online, online retail, uh, organization based in Hamburg. And from there I moved to Australia where I worked for CSIO, the [00:04:00] Commonwealth Science and Engineering Research, um, organization.
Habiba: It's, uh, and I worked as a research engineer helping with, uh, working with different, uh, government agencies in building machine learning and deep learning based methods for different applications. From there, I moved to Microsoft as an AI specialist and I worked with customers around Australia and New Zealand, helping them build their machine learning and data science solutions on Azure Cloud using Azure Cloud Services.
Habiba: And now I work as a data scientist at Department of Education in New South Wales. So here I am building different tools for the for in the for school staff and and students to to improve the education quality. So yeah, this is my, uh, your overall journey. And of course, like, uh, in as part of, uh, also I had been the CDF metric for the last two and a half years.
Habiba: So, you know, that's something I've been working on the site as well. Now, uh, my journey from academia to, um, to, uh, to industry, uh, the way it happened was that I was [00:05:00] always interested in trying to, uh, uh, work on unique and, uh, unique problems. So academia gave me that opportunity, especially research to help, uh, to understand.
Habiba: Different business problems and trying to find a very unique, customized solutions for those. And I realized that as a data scientist and a machine learning practitioner, uh, we need a lot of data and industry is, uh, with lots of application is a place where you can easily leverage a lot of techniques that you learn in research.
Habiba: So transitioned from, uh, from a pure academia to, uh, to industry where in a more applied machine learning settings and, uh, at metric as well. What inspired me was that we had, uh, we had a really unique challenges for small and medium sized businesses, how to help them grow their businesses, how to understand their numbers and how to help them, uh, understand how they can maintain and grow in a very competitive environment.
Habiba: So, um, I, there are very few solutions out [00:06:00] there which can help, which can give these kind of customized solution, uh, to the, uh, to these, uh, businesses. to small businesses. So that got me interested in, um, in, uh, in working on this application.
Mehmet: That's really, you know, like a very rich experience, I would say.
Mehmet: And, uh, you know, great, uh, also background that you have now, you know, from, you know, always, this is what I feel when, when someone, Um, is like very well versed in, in the technology side, especially in a domain, such machine learning and AI. So you are always on the hunt, as you mentioned, to, to find, you know, problem that you can solve with this use case.
Mehmet: And you choose, you know, like, finance management, which is, you know, part of a FinTech. So you just touched base, but I would love, you know, to know when did you feel, you know, like, yeah, like actually at Metric, you know, we are solving critical [00:07:00] challenges that SMEs and startups are facing. So if you can tell me or walk me through these challenges that they face usually and how, you know, applying machine learning, you know, is helping, you know, These customers to to get the results that they should be aiming for
Habiba: sure.
Habiba: So, um, the um The way the small businesses work is that they you know, they are very good with what they are trying to build Uh, but managing their finance is a different, uh, is it a completely different beast? Um, they usually um are Are very skilled in their craft But when they want to scale the, uh, they get into this challenging space, how to manage, you know, their, uh, what's, uh, what are they spending and what they are getting out of it.
Habiba: So, uh, and there are a number of tools out there, which they can leverage, which help them, you know, maintain their, uh, maintain their accounting. However, They are, um, they usually require [00:08:00] really in depth knowledge of accounting and, um, uh, and, uh, and is, are not so easy for a small business, which is, uh, you know, usually challenged by resources, uh, human resources and, uh, skills, uh, deficiency, as I said, they are skilled in what they are building, but, uh, you know, accounting requires some specialized, uh, knowledge and then they, and they get stuck with that and they, uh, you know, try to avoid doing that.
Habiba: And that, you know, uh, stops them from growing. So in that kind of scenario, they need something that can help them in a personalized way. Now, these personalized help are very expensive. So, you know, usually like you would need an accountant or a consultant or somebody with an expertise to, you know, help you understand your numbers and help you understand how you can grow.
Habiba: Now, this If you are a large organization, yes, you can hire people and you can, and they can help you with that. However, if you were a small business, you know, you don't have the, you can't afford, um, uh, extra support. So in that situation, [00:09:00] what can, uh, what can help you? So, um, this is where my inspiration started, uh, started that, you know, uh, I usually had been working with, uh, big corporate organizations with, you know, lots of funding and, you know, they are able to, like when they want to build something, they just, Uh, are able to hire the right people and, you know, build those things.
Habiba: But what happens when you are constrained, resource constrained? How do you make the most out of it? And AI and machine learning is one field where, uh, which is, uh, powered by data. So if you have data, you have the power and you, uh, it's just a matter of how do you leverage that data. And, uh, and so this is where, uh, I believe, uh, metric, you know, Uh, can, uh, can, uh, has a very special, um, position is in a very special position to help businesses because, uh, based on, uh, the, uh, the customer's own numbers, we can give them personalized, um, uh, metric of how their business is doing, what they can do to improve it.
Habiba: So, uh, and we don't, uh, and we can automate the process so [00:10:00] that, you know, they get this, uh, uh, this kind of feedback in real time, uh, which is something that, uh, you know, can help them, uh, feel empowered and grow their business.
Mehmet: You know, like you touched Habiba on a couple of things that I personally keep repeating that startups usually they don't have enough resources, they should be always and small businesses as well.
Mehmet: Um, they should be always on the hunt for products that, you know, um, can facilitate your touch base. Of course, you know, machine learning and AI, they are fantastic. I would say tools for us to be able to do more with less, as we can say, plus the automation part. Now, one thing which, uh, you know, as a CTO attracted me while preparing for, for this episode today, uh, you know, I've, I've seen in your bio, you mentioned like you have done thousands of conversations with customers.
Mehmet: And I love this because, you know, there's nothing that can first validate the idea better than talking to customers and then also shaping the product. So what, like, uh, where [00:11:00] the key insights and, you know, like the findings that you heard from the customers that also shaped, you know, through these conversations, uh, how you developed metric.
Habiba: Sure. So, um, this has also been, uh, you know, one of the experiences of learning for me as well as being the CEO of a startup that, uh, you know, I, uh, I had been working in one of the most advanced technologies, uh, that is AI and machine learning for a very long time. So you get really, um, uh, uh, charmed by, uh, by the, by the technique and you are interested in the methodology.
Habiba: But, uh, what, uh, what metric taught me and talking to customer taught me was, uh, that how do you, uh, uh, how do you, uh, use the technology for, uh, for the benefit of the end user? So it's not necessarily that, you know, you always have to have the fanciest tools, uh, to, uh, to work with. But what's important is that what is going to get the user, um, uh, you know.
Habiba: make things easier for the users. So, [00:12:00] for example, uh, one of the things that we are leveraging in metric is, um, uh, is, um, uh, is using OCR to help customer, um, you know, take pictures of the receipts and, you know, uh, get the data entry process, uh, uh, streamlined in a way that they don't have to, you know, do the manual entry.
Habiba: What that helps is that it saves their time. And also it helps us, uh, to process and give them output in a, in a more systematic way, uh, based on the outputs of the, uh, object, uh, character recognition kind of tools. So, um, so what I understood was that, uh, they didn't, you know, we, uh, we have to listen to the customer to understand what would, uh, what is their, uh, uh, what are their pain points and what can we.
Habiba: Practically due to, uh, uh, to answer those questions. So, um, so I think, yeah, like, uh, my, uh, multiple conversations and, you know, getting feedback from the customer is basically they want something that is easy and simple to use and is, uh, and is performant and is reliable. So these are the factors that, you know, [00:13:00] we take into consideration when we are trying to build something.
Habiba: And, uh, and so it doesn't has to be the most advanced techniques, but it just has to be something that works and is, uh, something that any user can trust.
Mehmet: I love this because you know, I'm big believer of listening to what the customers want. And then, and you know, you would agree with me, I think have you, but as a CTO and, you know, especially for you because you have built a lot of, uh, uh, products and systems also during your career.
Mehmet: So you have the business acumen and I think the finance is one of a kind. domain in technology that really the business acumen plays a lot of role in this. And this is why I want to ask you now, like I get to know that metric is used in over 70 countries and we know like especially when it comes to finance, legal, these kinds of stuff, things can be different from one country to another country.
Mehmet: So, um, Was that a challenge for you, like to learn about all [00:14:00] these, I would say, localizations needs? Or was it something that you, you know, you've done something, maybe using machine learning and AI to kind of automate this localization? If you can tell me a little bit more about, you know, because this is why I'm asking this question, because not only from technicality perspective, but Because I always tell startup funds at some stage, you start maybe in one country, let's be UAE, Saudi, Pakistan, Egypt, Turkey, but at some stage you need to scale out.
Mehmet: And then once you start to scale out, you need to have this understanding. Walk me through, you know, this journey of expanding to multiple geographies.
Habiba: Excellent question. Um, yeah, so this is something we are currently like trying to do. Um, so first of all, we are now in 180 plus countries now. Um, there are, I think, you know, 190 something countries in the world.
Habiba: And yeah, we surprisingly, uh, you know, organically we have, uh, um, people who have downloaded and, uh, at least tried, uh, our metric app, uh, in, [00:15:00] uh, in more, in over 180, uh, countries. Um, so, uh, but that's, you know, Those who have signed up, and then there's a different question of, you know, who are active users, etc, which is relatively limited.
Habiba: But, you know, we have quite a bit of presence organically in a number of countries. So, um, we, our journey started with Pakistan, you know, this was where we, um, it was our main customer base, and, you know, we were building it for small and medium sized businesses. Businesses in, uh, within Pakistan. But now that, uh, now we have expanded to, uh, to the Middle East and, uh, we are in UAE and, uh, you know, uh, uh, Saudi would be, uh, would be the next one.
Habiba: And, uh, and you know, then sky's the limit. So, uh, uh, you know, you, what you, uh, asked about, you know, like, uh, the, uh, mentioned about, you know, finite, uh, being a, a FinTech, uh, just that, you know, with different countries there are reg different regulations and Yeah. You know, like it's not one size fits all kind of a situation.
Habiba: So how do we deal with that? So, first of all, um. We are doing localization, uh, uh, one step at a time. Uh, and right now it's not, uh, something that we are leveraging AI or machine learning for. [00:16:00] But, uh, you know, in future when we wanna scale it to, you know, uh, to a more global market, we'll certainly be looking into solution that is, uh, uh, that is automated, that is, uh, machine learning based and that, and so that we don't have to do these, uh, uh, uh, uh, localizations, uh, one at a step at a time.
Habiba: So, uh, but our journey so far has been that, you know, uh, to, uh, to understand the regulations in each country and then, you know, build, uh, improve our product, uh, at, uh, at certain features in our products so that, uh, you know, from any country, uh, it is, uh, easy to and seamless to, uh, uh, for, uh, to, uh, integrate with, uh, with the business, local businesses.
Habiba: So anything from, you know, bank integration to, um, uh, to taxations, to any, uh, um, uh, to language localization. This is something that we are incorporating on our application, uh, uh, uh, one step at a time.
Mehmet: Fantastic. Now, the other thing I want to ask you, which is a little bit related to ai. Now of course, you are the expert here.
Mehmet: You know, I, I, I come from technology background, but not ai, [00:17:00] but of course I understand now recently when we say ai, have, I think you would agree with me, everyone. Mind goes to generative AI, right? So this is where people are going now. Of course, there's a huge potential over there. So first I would ask you, like, with the generative AI, everyone starts to say, and you mentioned the data also here, talk to your data.
Mehmet: So, so from this perspective, what are you seeing, you know, the, the benefits of coupling FinTech, especially with what you, what you do now at, uh, at Metric, with generative AI? But beyond this, I mean, beyond generative AI, how you see, you know, things moving forward, uh, in the domain of FinTech adopting other AI tools, because AGI is one tool, I mean, generative AI is just one tool.
Mehmet: So how you are seeing, uh, you know, other, Um, you know, like technologies within AI affecting the FinTech.
Habiba: Sure. So a few things, uh, that I'm seeing, and a few things that we are [00:18:00] already, um, you know, are, are working on, first of all is personalization. Uh, with the giving, uh, we are building a micro advisory for our end users.
Habiba: So the idea is that okay, you know, we are, um, uh, uh, you know, we are sitting on our customer's data and we, uh, our, one of our main, uh, goal is to provide insights. To the u to the end users based on their data and to help them and, and to give them tips and get to give them metrics and get to give them, um, uh, uh, some health score of how their business is doing and even doing some, uh, uh, some level of, uh, um, uh, um, of causality analysis that, what they need to do to improve their business.
Habiba: Or, you know, if like they're, uh, you know, what are the things that they had been doing in the past that can help, uh, that they were doing right and keep doing, or things that they can change, et cetera, not just based on their own business. But, uh, but generally, uh, generalizing it to more into like even their business sector or the region that they are operating in.
Habiba: So this has been something that we have been working on. Uh, but now with the generative ai, what we can, uh, uh, what we can easily, um, uh, add to the, to the [00:19:00] mix is that now a customer, now instead of us. pushing these, uh, these tips and feedback to the customer from our end. Uh, the customer can seek them themselves.
Habiba: So, you know, providing them with a conversational AI interface where they can ask questions about, you know, what do I need to do, how much should I be spending on marketing this, uh, this month? And, you know, uh, based on, uh, their data, We can give them insights as to, you know, uh, uh, what would work. Uh, so, uh, it could be based on their businesses or they are generally based on the, uh, generic sector that they are operating in.
Habiba: For example, if it's e commerce, so we can say that, you know, other, uh, generally organization in e commerce, they're spending in this, uh, area. And, you know, this is, you know, given you, you know, uh, what, uh, the, the revenue we have, this is, you know, how this percentage you should be spending on marketing or, you know, other, other areas.
Habiba: So this is, I think, you know, a very straightforward, um, uh, use case of an application of generative AI in this space. Now, uh, taking it further, what I see in future is where generative AI can be even more helpful [00:20:00] is, um, is giving a more visual outputs to the user. So, you know, not just responding, uh, in a, in a conversational interface, but also giving them, um, a visual output.
Habiba: Detailed graphs and charts and, you know, images off, you know, uh, our trends, industrial trends, etcetera. So I think, you know, this is and this is not something, you know, really too far in future. This is something people are already working on and can easily leverage. So I feel like, you know, that would make the whole process off.
Habiba: Um, uh, yeah. generating insights a lot easier and simpler. Uh, and, um, uh, and you know, um, this is something that, uh, uh, uh, that would, uh, empower the businesses even more.
Mehmet: That's, uh, you know, great to hear this, what's coming in the future. And I think, you know, you're leveraging, as you mentioned before, uh, actually your product is built around machine learning and AI.
Mehmet: So I'm sure like you would have a rich roadmap in the future. I'm, I'm right, right?
Habiba: Yep. Yeah, totally. [00:21:00] Yeah, this is something our main focus, uh, moving forward.
Mehmet: Great. Now, I want to shift a little bit, Giyus, because, you know, like, a lot of people are interested now to be in this domain, you know, and we know, like, the base for machine learning and everything that comes around it.
Mehmet: To your point, again, we go back to the data. And now, um, you know, people are thinking how we can get into this domain. So by being, for example, Starting being a data scientist, right? So what advice you would give to young professionals and students, mainly who they, you know, they want to pursue a career in this domain in that data science, machine learning?
Mehmet: Um, and maybe, you know, if you tell us also within that domain, like, should they also, I mean, not necessarily one domain, but are there like, you know, Any applications similar to FinTech that they can also focus on that can niche down to that?
Habiba: Great. Yes. So, um, so my first advice [00:22:00] is that, you know, given that, you know, the way AI has evolved in the last, uh, just last one year, um, uh, I feel like, um, getting into AI and machine learning, it has become a lot easier, uh, because now, um, there are, So many prebuilt powerful models that you can just leverage.
Habiba: So, you know, you basically need to have a little bit of engineering background to know how to, you know, call an API and build a front end interface to, uh, to, uh, to, you know, get started. So it was, uh, it was cool. Quite different. Uh, a few years ago when to get into this, uh, this field, you would mostly have to try to build your own models.
Habiba: You know, you would have to train your own models and, you know, deploy it somewhere and then use it. Um, and this would require, you know, some specialized skills. I'm learning of, you know, how do you build these models? And, you know, where do you deploy them? Law, et cetera, et cetera. Now, uh, with these prebuilt models, it's just that, you know, you, uh, need to have, uh, a certain level of understanding of how you, you know, you call APIs, you know, from different tools and technologies [00:23:00] and, uh, how to use them.
Habiba: So I think it has made it a lot easier. What I find is that, uh, still, um, uh, a big gap is, uh, the challenge of finding a good business problem that can be used, uh, uh, uh, that AI or machine learning, or these, uh, large, uh. Uh, language models are generative. I can actually, uh, help answers those questions. So I think, you know, um, there's a lot of hype.
Habiba: There's a lot of excitement, but you know, practically what you know, they can achieve what their limitations are something that you know, are something that we have to be very careful, especially when you talk about fintech, you know, you mentioned, you know, the highly regulated, uh, industry and, uh, and, you know, with working with sensitive data.
Habiba: So we need to be very careful that, you know, how we, um, using any prebuilt model. We What is exactly it's outputting and how do we, uh, do the evaluation of, you know, the, the responses it's providing us, uh, are, uh, are actually useful. So how do you, uh, how do we measure that? So, you know, we, this is something we need to be, uh, to be careful about now, which fields are the one that I feel, uh, where, [00:24:00] uh, these techniques can be very, very useful.
Habiba: Other than FinTech is, uh, what I found is that in health sector, uh, in, uh, and in marketing, these, uh, tools are heavily used. Used and they are, um, uh, uh, and they, they are very use, uh, u uh, useful. Uh, the reason I find, uh, them, uh, potentially very useful in those industries is because of the, um, uh, of the, of the data that is, uh, that is available to fine tune these models.
Habiba: So, you know, you have these base base models, foundation models, but then, uh, for every application you have to customize to a, to a, to, to, uh, to. So you need some kind of fine tuning so that it's really is able to answer the kind of questions, uh, which is very specific to your domain. And I feel like, uh, you know, uh, uh, in healthcare and marketing, we do have those sort of data that can be used to help find you.
Habiba: And also, uh, you know, how you can, uh, uh, I think the, uh, very, um, Uh, A big differentiator of using these models is when you, uh, when you use your, [00:25:00] uh, when you, um, uh, use a proprietary data to, uh, to, uh, to answer not just a generic question, but, you know, specific to a particular domain and for your users. So I think, you know, this technique called, um, RAG retrieval augmented generation is something that is, uh, definitely going to be, uh, something, uh, leveraged a lot in future.
Mehmet: Absolutely. And, you know, I remember when I tried one time to, you know, to just out of curiosity to see how this machine learning works. And, you know, I came across one course and there was like, they touched base on like something like deep learning tensorflow and these, you know, libraries. And, you know, like, just like matter of training, little bit of data, it's huge effort.
Mehmet: And now we are lucky enough to have it. you know, big companies build these large language models and you know, that are ready for us to use, like even myself without like, I would say proper, uh, data science background. Of course, I cannot do everything that a data scientist would [00:26:00] do, but I mean, for me, as a normal user, I can, you know, get the data, manipulate the data and do do useful stuff with it.
Mehmet: So, so this is really amazing. And to your point, a lot of guests, mentioned health tech, another area, uh, which is very promising education also as well. You know, I'm personally excited about that area as well. So definitely now, um, you know, like it's kind of a traditional question I ask, but, and you just mentioned many things change in one year.
Mehmet: You're absolutely right. But you know, what are the trends that we should be expecting AI and machine learning? And I will not tell you in a couple of years, I would just tell you in the near future, maybe 12 months or so. So what are your expectations?
Habiba: So, um, a few trends that I can see coming in future is, uh, you know, uh, I think from a hardware perspective, I feel like, you know, things, uh, uh, you know, uh, the availability of, uh, uh, of, uh, powerful compute so that you can train your own models.
Habiba: I think this is something that is, uh, lacking. Uh, but I [00:27:00] think, uh, they, uh, definitely, uh, now there is a big need for it now that we know, uh, the power of these, uh, large language models. So, uh, more and more companies are trying to build their own custom solutions. And for that you need compute. So I think, you know, uh, from a, uh, not necessarily from a software technology or the methodology perspective of more like a, you know, like having a cheaper, uh, hardware available.
Habiba: I mean, that, that, that's how even large line models came about, you know, the, uh, um, the basic concepts were still there. So natural language processing has been there for a very long time. And, you know, in deep learning, we were, uh, already building deep learning models. But you know, what's the, what is the difference that these generated by a models are bringing is just that they are huge.
Habiba: They have billions of parameters and they are leveraging a lot of data and to leverage a lot of data. You need a, you need a powerful compute to be able to train like a deep model with billions of parameters. Again, you need compute. So the basic, uh, uh, methodology was, you know, still there. But, you know, what made it, [00:28:00] you know, made the whole difference was, you know, having, uh, being able to train these large models.
Habiba: So I think, you know, what I see in the next 12 or so months is, and this is something I've been seeing at my, uh, in, uh, with, uh, with anyone I'm working with that they are just, you know, waiting, uh, until, you know, they can train their own models so that, you know, they have more power, uh, they have more control over, uh, What they are, you know, building and not just using, you know, these pre built models that are black boxes because because I feel like You know, the more we get into it, we have to be careful from a responsible AI perspective that what is, uh, this, uh, how, how much we can trust the output of these models.
Habiba: I mean, you know, in the end, they're in the end. In the end, they're all stochastic models. So they give as exact answer as possible. you know, based on the data you're, uh, they're, uh, they're trained on. So depending on, you know, what your sources of data is, uh, you know, they have their, uh, biases built into it.
Habiba: And hallucination is another issue that, you know, is well known about large language models that, you know, they are, uh, [00:29:00] sometimes, you know, like, uh, having learned through, uh, such a huge corpus of data, They start becoming very creative and you know, the responses may not be, you know, useful in the sense that you know, they are, they're exact.
Habiba: So, you know, these are the places where I feel like, you know, there will be a lot more work done. So one is, you know, from a hardware computational perspective, then secondly, responsible AI and then evaluating the, uh, the These large engine models. So even I get challenged by, you know, seeing like it's overwhelming that, you know, pretty much like every day there are new, uh, foundational models that, uh, that you, uh, you hear about and everyone would claim that their models are the best, but how do you evaluate them?
Habiba: How do you benchmark these different technologies? So I think, you know, uh, there will be a lot more work done in, uh, in that, uh, in that area in near future.
Mehmet: Absolutely. And, you know, of course the first part, you know, I think everyone would agree with you about, you know, and everyone, you know, talking about how I can train and, you know, have this compute locally on my machine, even on my [00:30:00] smartphone.
Mehmet: Uh, I know like Samsung, they're trying to do something. I know Apple. They are trying to do something also as well in that, uh, and other, I mean, hardware vendors as well. And the second point, this is why we start to see and, you know, like I never seen, you know, when you have a technology and then a technology that compliment very fast.
Mehmet: So everyone is talking about, uh, you know, retrieval augmented generation, which is a rag, you know, they call it, you know, just to, to make sure that they are, is more accurate, more, uh, uh, uh, To your point, it's not hallucinating. There's a lot of startups also in that domain, which is fascinating because you know, like we just have the generative AI and then just less than one year, we start to have these complimentary startups.
Mehmet: Now, as we're coming almost to an end, happy about with you today. Uh, final advice from you also for you. People who want to start, I mean, to go and have a startup, especially if they come from a technology domain, what you can tell them and where we can find more and [00:31:00] learn more about you and metric app.
Habiba: Sure. So, um, I think, you know, um, what I would, my advice would be to, um, to, uh, to start, uh, small and, uh, um, uh, and, and build and re, uh, and iterate over it. Okay. So instead of, uh, you know, for, in a, in a startup world and the way, you know, things, especially in the tech side and AI side, things are changing so rapidly that you cannot sit around, uh, for six months, uh, trying to build one feature or one, uh, uh, uh, one tool you need to do it, uh, you need to be, uh, you know, experiment, uh, quickly, uh, and then iterate over it.
Habiba: So we talked about in the very beginning about, you know, getting feedback from our customers, from the customers. So this is, uh, really important that, you know, start, uh, start building, See, uh, see, get, um, uh, input from the, uh, from the end user, how they feel about it and iterate over it. Um, I feel like building things is, uh, has become a lot easier.
Habiba: The challenging part is to find your niche, find that, you know, where you can, [00:32:00] uh, add the value, uh, the value you can make a, be a differentiator. So, you know, um, I hear pretty much like every day. You know, there are a lot of startups that are, you know, working in this generative AI space, as you mentioned as well, that yes, you know, literally, like every day you would hear about, you know, a new startup doing that.
Habiba: But then, uh, in this competitive environment, environment, you need to understand that it's not just about, you know, knowing the technology, it's just about how to use it properly and where it will, uh, Uh, uh, add some value. So I think, you know, uh, and for that you need constant feedback. So, you know, uh, start small, build things, uh, uh, see how it goes and, uh, and uh, and improve on it.
Habiba: So I think, yeah, this is something really important.
Mehmet: Great. And what's the, any website where we can find about metric and, you know, anything about you, Habi.
Habiba: Uh, sure. Uh, so for the metric, uh, you know, our, our, our website metric app.ai, uh, is where, uh, you can, uh, find out, uh, what we are, uh, our products and what we are, uh, what we have built, and, you know, uh, where we are, we are headed.
Habiba: Um, and you can find me on [00:33:00] LinkedIn at, uh, Habiba do h, so H-A-B-I-B-H, and, uh, um, sorry, Habiba dash H, not uh, h Uh, so yeah, I am, uh, uh, connect with me over there and, uh, you know, if you have any questions, I'll be happy to answer.
Mehmet: Sure. Thank you very much. I will make sure that, you know, I will put the links in the show notes and really thank you very much.
Mehmet: And just as a, you know, small feedback on what you mentioned last, this is, you know, my, as they say, my two cents for fellow founders. You mentioned previously in one of the answers for my questions about finding The business problem and then build the solution on it and then go and start and get feedback.
Mehmet: So absolutely 100 percent I agree with you happy. But thank you very much for being with me today on this episode. I really appreciate the time and all the knowledge and, you know, also congratulations on metric habit, you know, fantastic technology that it Put in the hands of uh startups and small medium businesses that they need the most support from us So thank you for that.
Mehmet: And this is how [00:34:00] I end usually my episodes. So this is for the audience If you just discovered this podcast by like thank you for passing by if you like it Please subscribe and share it with your friends and colleagues and you know family. And if you are one of the, uh, people who keep coming, the loyal followers, thank you very much for your feedback.
Mehmet: Thank you very much for your suggestions. Keep them coming. And I'm hoping that you are enjoying this podcast. If you do so also don't forget to share it with your friends and colleagues. Thank you very much for tuning in. We'll meet again very soon. Thank you. Bye. Bye.
Habiba: Thank you Mehmet.